Artificial Intelligence (AI) Lab
The British Antarctic Survey’s Artificial Intelligence (AI) Lab is at the forefront of innovative research and innovation, employing AI and machine learning techniques to tackle a broad spectrum of challenges within the institute’s remit. From sea ice forecasting and space weather, ice dynamics and sea level rise, seasonal polar operations and automation, to tracking wildlife and icebergs from space and benthic biology on the seafloor.
Extracting knowledge from the diverse range of datasets and domain areas which span various spatial and time scales poses significant challenges. The BAS AI Lab leverages the power of AI to intelligently integrate different types of data to pave the way for new discoveries and unlocking previously inaccessible information.
One of the AI Lab’s focuses is optimising data collection processes in remote and hostile environments to reduce carbon emissions and maximise science output. By integrating satellite observations, surface sensors, and optimising task planning for infrastructure and logistics (including ships and autonomous marine vehicles) the AI Lab strives to streamline data acquisition and improve the efficiency of scientific expeditions. This approach ensures that valuable resources are used sustainably, minimising carbon footprint.
![]() |
![]() |
![]() |
![]() |
![]() |
Research areas within the BAS AI Lab Click to see more ! |
As a leading national entity, BAS’s AI Lab actively collaborates with national and international partners to co-develop and co-deliver AI methods that are tailored to meet the unique requirements of polar research. By working closely with experts from diverse backgrounds, the lab ensures that the developed AI techniques are fit for purpose and aligned with global standards to enable wider adoption.
An important aspect of the AI Lab’s mission is to put novel, freely available digital solutions into the hands of decision-makers. By leveraging the power of AI, the lab creates operational tools and technologies that aid policymakers and stakeholders in making informed decisions to safeguard our planet. These solutions provide valuable insights and enable proactive mitigation and adaptation measures to help address the climate and biodiversity crisis.
PhD opportunities
- Centre for Doctoral Training (CDT) in the Application of Artificial Intelligence to the study of Environmental Risks, University of Cambridge and British Antarctic Survey
IceNet
IceNet is a probabilistic, deep learning sea ice forecasting system developed by an international team and led by British Antarctic Survey and The Alan Turing Institute [Andersson et al., 2021]. …AI for smart conservation
In the AI for smart conservation project, BAS are collaborating with local ecologists and conservation agencies to develop decision-making tools informed by sea ice forecasts. By combining satellite observations, GPS …AI for Earth Observation
Recent increases in the spatial coverage and temporal resolution of 40 m resolution Synthetic Aperture Radar (SAR) imagery, is opening new opportunities to rapidly detect environmental observations e.g. ice sheet and iceberg position with greater positional accuracy. Our team are developing supervised and unsupervised machine learning techniques to automatically detect sea ice and iceberg position from Sentinel-1 SAR imagery.Digital Twins of the Polar Regions
Digital Twinning is next generation technology for data fusion and computer modelling enabling us to rapidly get answers to “what-if” questions. Digital Twins (DTs) are already in operation in industry …DI4EDS
Environmental research relies on digital infrastructure (hardware, software and methods) to provide services that help researchers answer questions about the environment around us, and innovators to work out ways that …Using AI to track icebergs
23 November, 2023
Researchers are using a new AI tool to detect icebergs in the Southern Ocean. This is the first step towards scientists being able to track the complete life cycle of …
AI tool to revolutionise polar ship navigation
15 November, 2022
Artificial Intelligence (AI) will enable ships navigating in polar ocean conditions to be more efficient using a new route planning tool created by British Antarctic Survey (BAS) researchers. The tool …
Space technology and artificial intelligence to monitor whale mass stranding events
18 November, 2021
An international team of scientists led by British Antarctic Survey have published research today on using new technology to study mass stranding of whales from space and how the technology …
Artificial intelligence to help predict Arctic sea ice loss
26 August, 2021
A new AI (artificial intelligence) tool is set to enable scientists to more accurately forecast Arctic sea ice conditions months into the future. The improved predictions could underpin new early-warning …
Using AI to track whales from space
4 February, 2021
British Antarctic Survey (BAS) scientists will work with an Artificial Intelligence company after being awarded a contract from the Canadian Space Agency (CSA) to support the protection of an endangered …
PhD centre will nurture new leaders in Earth observation
9 January, 2020
A new centre will enable 50 fully-funded PhD researchers to harness satellite data to tackle global environmental challenges. The Centre for Satellite Data in Environmental Science (SENSE) will bring together expertise in …
Using AI to help tackle global environmental challenges
26 February, 2019
A new Centre for Doctoral Training, involving researchers from British Antarctic Survey, will develop Artificial Intelligence (AI) techniques to address critical environmental challenges. Climate change and environmental hazards pose some …
Watching whales from space
1 November, 2018
Scientists have used detailed high-resolution satellite images provided by Maxar Technologies’ DigitalGlobe, to detect, count and describe four different species of whales. Reported this week in the journal Marine Mammal …
Seeing double: Digital twins and net zero
5 July, 2022 by Jonathan Smith
Reaching net zero, as a country or a business, requires new measures, technology and innovations. Digital twins are an example of this; they can be a powerful tool to drive innovation and efficiency.
BLOG: Predicting September 2021 Arctic sea ice using artificial intelligence
24 September, 2021 by Tom Andersson
Tom Andersson, a data scientist at British Antarctic Survey Artificial Intelligence (AI) Lab, shares the latest predictions from a new Arctic sea ice forecasting AI tool as this year’s Arctic …
Innovative Research Sprints Tackle Challenges in Biodiversity and Exposure
11 May, 2021 by Anita Faul
Over the last decade, digital technologies, including Artificial Intelligence, developed rapidly as did our capability to monitor our home planet from space with Earth Observation satellites. How can we most …
Earth Day 2020: A new age of Arctic science discovery – the AI way
24 April, 2020 by Scott Hosking
When we see news reports on climate change on our TV, they are often accompanied by footage of a polar bear walking over the icy Arctic landscape. But the Arctic …
Official launch of the BAS AI Lab!
4 December, 2019 by Risa Ueno
Today we officially launched the BAS AI Lab – thank you everyone for coming!
AI Lab presents at the First Artificial Intelligence for Copernicus Workshop
22 November, 2019 by Anita Faul
Andrew Fleming and Anita Faul present their research at the First Artificial Intelligence for Copernicus Workshop
Machine Learning for Environmental Sciences
21 July, 2019 by Rachel Furner
Rachel Furner is a PhD student at British Antarctic Survey, which has recently opened up its new AI Lab, that aims to foster the application of various machine learning (and …
Data Study Group: Automated monitoring of seals via high-resolution satellite imagery
8 April, 2019 by Premdeep Gill
Prem hosted at Data Study Group at the Alan Turing Institute Seals from space: automated Antarctic ecosystem monitoring via high-resolution satellite imagery Antarctic seal populations are potential indicators for the …
Automated clustering of storm tracks for interpreting ice core records
22 August, 2017 by Scott Hosking
Accumulation in coastal West Antarctic ice core records and the role of cyclone activity Cyclones are an important component of Antarctic climate variability, yet quantifying their impact on the polar …
Exploring the dynamics of Lotka–Volterra systems: Efficiency, extinction order, and predictive machine learning
3 March, 2025 by Michael Thorne
For years, a main focus of ecological research has been to better understand the complex dynamical interactions between species which comprise food webs. Using the connectance properties of a widely…A polar oceans shipping information system
1 February, 2025 by Andrew Fleming, Alexander Tate, Andreas Cziferszky, Jeremy Wilkinson, Michael Thorne
Globally, ships above a certain tonnage, as well as an increasing number of smaller vessels, rely on the AIS (Automatic Identification System) to safely navigate around other vessels, which are…Calculations of extreme sea level rise scenarios are strongly dependent on ice sheet model resolution
28 January, 2025 by Rosie Williams, James Byrne, Scott Hosking, Robert Arthern
The West Antarctic Ice Sheet (WAIS) is losing ice and its annual contribution to sea level is increasing. The future behaviour of WAIS will impact societies worldwide, yet deep uncertainty…Surveying the deep: A review of computer vision in the benthos
22 January, 2025 by Cameron Trotter, Huw Griffiths, Rowan Whittle
The analysis of image data for benthic biodiversity monitoring is now commonplace within the domain of marine ecology. Whilst advances in imaging technologies have allowed for the collection of vast…Read more on Surveying the deep: A review of computer vision in the benthos
Improving the reproducibility in geoscientific papers: lessons learned from a Hackathon in climate science
16 January, 2025 by Andrew McDonald, Scott Hosking
In this paper, we explore the crucial role and challenges of computational reproducibility in geosciences, drawing insights from the Climate Informatics Reproducibility Challenge (CICR) in 2023. The competition aimed at…The challenge of land in a neural network ocean model
2 January, 2025 by Dave Munday, Dani Jones, Rachel Furner
Machine learning (ML) techniques have emerged as a powerful tool for predicting weather and climate systems. However, much of the progress to date focuses on predicting the short-term evolution of…Read more on The challenge of land in a neural network ocean model
Machine learning for stochastic parametrization
2 January, 2025 by Raghul Parthipan
Atmospheric models used for weather and climate prediction are traditionally formulated in a deterministic manner. In other words, given a particular state of the resolved scale variables, the most likely…Read more on Machine learning for stochastic parametrization
Streamflow prediction using artificial neural networks and soil moisture proxies
1 January, 2025 by Scott Hosking
Machine learning models have been used extensively in hydrology, but issues persist with regard to their transparency, and there is currently no identifiable best practice for forcing variables in streamflow…Read more on Streamflow prediction using artificial neural networks and soil moisture proxies
Investigating the Impact of Feature Reduction for Deep Learning-based Seasonal Sea Ice Forecasting
1 January, 2025 by Andrew McDonald, Scott Hosking
With the state-of-the-art IceNet model, deep learning has contributed to an important aspect of climate research by leveraging a range of climate inputs to provide accurate forecasts of Arctic sea…Downscaling precipitation over High-mountain Asia using multi-fidelity Gaussian processes: improved estimates from ERA5
18 November, 2024 by Andrew Orr, Scott Hosking, Kenza Tazi
The rivers of High-mountain Asia provide freshwater to around 1.9 billion people. However, precipitation, the main driver of river flow, is still poorly understood due to limited in situ measurements…Contributions to the development of the next-generation NERC Environmental Data Service: Building Interoperability – a NERC Data Commons RoadMap
1 November, 2024 by Alexander Tate, Alice Fremand, Helen Peat, James Byrne, Petra Ten Hoopen
This is a final report from the work undertaken by the NERC Environmental Data Service (EDS) funded by the UKRI Digital Research Programme grant: EDS UKRI DRI Phase 1b. The…The AutoICE Challenge
7 August, 2024 by Martin Rogers
Mapping sea ice in the Arctic is essential for maritime navigation, and growing vessel traffic highlights the necessity of the timeliness and accuracy of sea ice charts. In addition, with…Multi-population Evolutionary and Swarm Intelligence Dynamic Optimization Algorithms: A Survey
17 July, 2024 by Donya Yazdani
Multi-population evolutionary and swarm intelligence dynamic optimization algorithms are the most flexible and effective methods for solving dynamic optimization problems. In a dynamic optimization problem, the search space is affected…Sea ice detection using concurrent multispectral and synthetic aperture radar imagery
1 May, 2024 by Andrew Fleming, Scott Hosking, Jeremy Wilkinson, Maria Fox, Martin Rogers
Synthetic Aperture Radar (SAR) imagery is the primary data type used for sea ice mapping due to its spatiotemporal coverage and the ability to detect sea ice independent of cloud…Read more on Sea ice detection using concurrent multispectral and synthetic aperture radar imagery
WAVI.jl: Ice Sheet Modelling in Julia
14 March, 2024 by Alexander Bradley, Rosie Williams, David Bett, James Byrne, Robert Arthern
Ice sheet models are used to improve our understanding of the past, present, and future evolution of ice sheets. To do so, they solve the equations describing the flow of…Interrogating Sea Ice Predictability with Gradients
14 February, 2024 by Scott Hosking
Predicting sea ice concentration is an important task in climate analysis. The recently proposed deep learning system IceNet is the state of the art sea ice prediction model. IceNet takes…Read more on Interrogating Sea Ice Predictability with Gradients
Review of Satellite Remote Sensing and Unoccupied Aircraft Systems for Counting Wildlife on Land
8 February, 2024 by Ellen Bowler, Hannah Cubaynes, Marie Attard, Penny Clarke, Peter Fretwell, Richard Phillips
Although many medium-to-large terrestrial vertebrates are still counted by ground or aerial surveys, remote-sensing technologies and image analysis have developed rapidly in recent decades, offering improved accuracy and repeatability, lower…Prioritize environmental sustainability in use of AI and data science methods [Comment]
26 January, 2024 by Scott Hosking
Artificial Intelligence (AI) and data science will play a crucial role in improving environmental sustainability, but the energy requirements of these methods will have an increasingly negative effect on the…Read more on Prioritize environmental sustainability in use of AI and data science methods [Comment]
Unsupervised machine learning detection of iceberg populations within sea ice from dual-polarisation SAR imagery
1 November, 2023 by Andrew Fleming, Anita Faul, Ben Evans, David Vaughan, Scott Hosking
Accurate quantification of iceberg populations is essential to inform estimates of Southern Ocean freshwater and heat balances as well as shipping hazards. The automated operational monitoring of icebergs remains challenging,…Histopathological screening of Pontogammarus robustoides (Amphipoda), an invader on route to the United Kingdom
1 September, 2023 by Martin Rogers
Biological invasions may act as conduits for pathogen introduction. To determine which invasive non-native species pose the biggest threat, we must first determine the symbionts (pathogens, parasites, commensals, mutualists) they…
AI Lab Leadership
- Scott Hosking (Leader)
- Martin Rogers (Deputy)
- Jonathan Smith (Deputy)
Share this
Featured
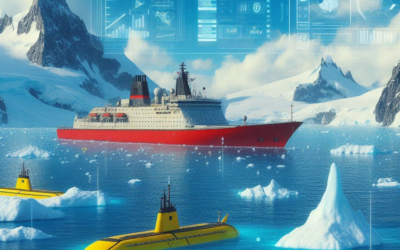
AMOP – Autonomous Marine Operations Planning
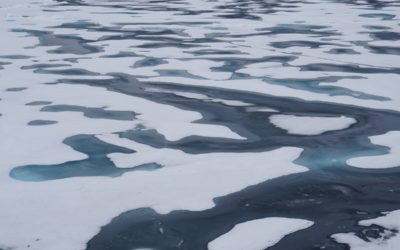